Introduction to the Gene Expression Analysis
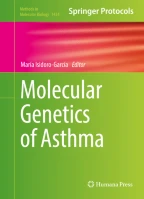
In 1941, Beadle and Tatum published experiments that would explain the basis of the central dogma of molecular biology, whereby the DNA through an intermediate molecule, called RNA, results proteins that perform the functions in cells. Currently, biomedical research attempts to explain the mechanisms by which develops a particular disease, for this reason, gene expression studies have proven to be a great resource. Strictly, the term “gene expression” comprises from the gene activation until the mature protein is located in its corresponding compartment to perform its function and contribute to the expression of the phenotype of cell.
The expression studies are directed to detect and quantify messenger RNA (mRNA) levels of a specific gene. The development of the RNA-based gene expression studies began with the Northern Blot by Alwine et al. in 1977. In 1969, Gall and Pardue and John et al. independently developed the in situ hybridization, but this technique was not employed to detect mRNA until 1986 by Coghlan. Today, many of the techniques for quantification of RNA are deprecated because other new techniques provide more information. Currently the most widely used techniques are qPCR, expression microarrays, and RNAseq for the transcriptome analysis. In this chapter, these techniques will be reviewed.
This is a preview of subscription content, log in via an institution to check access.
Access this chapter
Subscribe and save
Springer+ Basic
€32.70 /Month
- Get 10 units per month
- Download Article/Chapter or eBook
- 1 Unit = 1 Article or 1 Chapter
- Cancel anytime
Buy Now
Price includes VAT (France)
eBook EUR 85.59 Price includes VAT (France)
Softcover Book EUR 105.49 Price includes VAT (France)
Hardcover Book EUR 105.49 Price includes VAT (France)
Tax calculation will be finalised at checkout
Purchases are for personal use only
Similar content being viewed by others
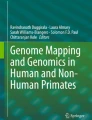
Gene Expression Studies and Complex Diseases
Chapter © 2015
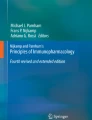
Gene Expression
Chapter © 2019
Tissue Specificity of Gene Expression
Article 29 September 2016
References
- Saiki RK, Scharf S, Faloona F et al (1985) Enzymatic amplification of beta-globin genomic sequences and restriction site analysis for diagnosis of sickle cell anemia. Science 230:1350–1354 ArticleCASPubMedGoogle Scholar
- Mallona I (2008) Selección de genes de normalización para RT-PCR cuantitativa en Petunia hybrida. (Normalization gene selection for quantitative RT-PCR in Petunia hybrida). Available via http://repositorio.bib.upct.es/dspace/handle/10317/723. Accessed 25 Nov 2014
- Higuchi R, Dollinger G, Walsh PS et al (1992) Simultaneous amplification and detection of specific DNA sequences. Biotechnology 10:413–417 ArticleCASPubMedGoogle Scholar
- Higuchi R, Fockler C, Dollinger G et al (1993) Kinetic PCR analysis: real-time monitoring of DNA amplification reactions. Biotechnology 11:1026–1030 ArticleCASPubMedGoogle Scholar
- Clewley JP (1994) The polymerase chain reaction (PCR) for human viral diagnosis. CRC Press, Boca Ratón Google Scholar
- Taylor S, Wakem M, Dijkman G et al (2010) A practical approach to RT-qPCR-Publishing data that conform to the MIQE guidelines. Methods 50:S1–S5 ArticleCASPubMedGoogle Scholar
- Huggett J, Bustin S (2011) Standardization and reporting for nucleic acid quantification. Accred Qual Assur 16:399–405 ArticleCASGoogle Scholar
- Kozera B, Rapacz M (2013) Reference genes in real-time PCR. J Appl Genet 54:391–406 ArticleCASPubMedPubMed CentralGoogle Scholar
- Zipper H, Brunner H, Bernhagen J et al (2004) Investigations on DNA intercalation and surface binding by SYBR Green I, its structure determination and methodological implications. Nucleic Acids Res 32(12):e103 ArticlePubMedPubMed CentralGoogle Scholar
- Sigma-Aldrich (2008) qPCR Technical Guide. Available via http://www.sigmaaldrich.com/content/dam/sigma-aldrich/docs/Sigma/General_Information/qpcr_technical_guide.pdf. Accessed 22 Nov 2014
- http://www.gene-quantification.de/hrm-dyes.html
- VanGuilder HD, Vrana KE, Freeman WM (2008) Twenty-five years of quantitative PCR for gene expression analysis. Biotechniques 44:619–626 ArticleCASPubMedGoogle Scholar
- Qiagen (2006) Critical factors for successful real time PCR. Integrated solutions-real time PCR applications. Available via http://jornades.uab.cat/workshopmrama/sites/jornades.uab.cat.workshopmrama/files/Critical_factors_successful_real_time_PCR.pdf. Accessed 28 Nov 2014
- Leonard DGB (2007) Molecular pathology in clinical practice. Springer Science & Business Media, Berlin BookGoogle Scholar
- Bustin SA, Kessler HH (2010) Amplification and detection methods. In: Kessler HH (ed) Molecular diagnostics of infectious diseases. De Gruyter, Berlín Google Scholar
- Louw TM, Booth CS, Pienaar E et al (2011) Experimental validation of a fundamental model for PCR efficiency. Chem Eng Sci 66:1783–1789 ArticleCASPubMedPubMed CentralGoogle Scholar
- Diez GO (2006) Técnicas de Genética Molecular II (Molecular Genetic Techniques II). In: Lasa A (ed) PCR cuantitativa (quantitative PCR). SEQC, Barcelona Google Scholar
- Livak KJ, Schmittgen TD (2001) Analysis of relative gene expression data using real-time quantitative PCR and the 2(-Delta Delta C(T)) method. Methods 25:402–408 ArticleCASPubMedGoogle Scholar
- Pfaffl MW (2001) A new mathematical model for relative quantification in real-time RT-PCR. Nucleic Acids Res 29:2002–2007 ArticleGoogle Scholar
- Pfaffl MW (2004) Quantification strategies in real-time PCR. In: Bustin SA (ed) A-Z of quantitative PCR. International University Line, La Jolla Google Scholar
- Bohla L, Dusanic D, Narat M et al (2012) Comparison of methods for relative quantification of gene expression using real-time PCR. Acta Agric Slov 100:97–106 Google Scholar
- Mallona I, Lischewski S, Weiss J et al (2010) Validation of reference genes for quantitative real-time PCR during leaf and flower development in Petunia hybrida. BMC Plant Biol 10:4 ArticlePubMedPubMed CentralGoogle Scholar
- Bustin SA, Benes V, Garson JA et al (2009) The MIQE guidelines: minimum information for publication of quantitative real-time PCR experiments. Clin Chem 55:611–622 ArticleCASPubMedGoogle Scholar
- Valente V, Teixeira SA, Neder L et al (2009) Selection of suitable housekeeping genes for expression analysis in glioblastoma using quantitative RT-PCR. BMC Mol Biol 10:17 ArticlePubMedPubMed CentralGoogle Scholar
- Podevin N, Krauss A, Henry I et al (2012) Selection and validation of reference genes for quantitative RT-PCR expression studies of the non-model crop Musa. Mol Breed 30:1237–1252 ArticleCASPubMedPubMed CentralGoogle Scholar
- Fu W, Xie W, Zhang Z et al (2013) Exploring valid reference genes for quantitative real-time PCR analysis in Plutella xylostella. Int J Biol Sci 9:792–802 ArticlePubMedPubMed CentralGoogle Scholar
- Gantasala NP, Papolu PK, Thakur PK et al (2013) Selection and validation of reference genes for quantitative gene expression studies by real-time PCR in eggplant (Solanum melongena L). BMC Res Notes 6:312 ArticleCASPubMedPubMed CentralGoogle Scholar
- Paim RM, Pereira MH, Di Ponzio R et al (2012) Validation of reference genes for expression analysis in the salivary gland and the intestine of Rhodniusprolixus (Hemiptera, Reduviidae) under different experimental conditions by quantitative real-time PCR. BMC Res Notes 5:128 ArticleCASPubMedPubMed CentralGoogle Scholar
- Tunbridge EM, Eastwood SL, Harrison PJ (2011) Changed relative to what? Housekeeping genes and normalization strategies in human brain gene expression studies. Biol Psychiatry 69:173–179 ArticleCASPubMedGoogle Scholar
- Southern E, Mir K, Schepinov M (1999) Molecular interactions on microarrays. Nat Genet 21:5–9 ArticleCASPubMedGoogle Scholar
- Eberwine JH, Valentino KL, Barchas JD (1994) In situ hybridization in neurobiology: advances in methodology. Oxford University Press, Oxford Google Scholar
- McLachlan G, Do K, Ambroise C (2005) Analyzing microarray gene expression data. Wiley, Hoboken Google Scholar
- Faiz A, Burgess JK (2012) How can microarrays unlock asthma? J Allergy 2012:241314 ArticleGoogle Scholar
- Affymetrix (2002) Affymetrix, Stanford University and incyte resolve patent oppositions and interferences. Available via http://investor.affymetrix.com/phoenix.zhtml?c=116408&p=irol-newsArticle_pf&ID=362094. Accessed 21 Dec 2014
- Times Higher Education (2006) Background memo on the winners of the European inventor of the year 2006 awards. Available via http://www.timeshighereducation.co.uk/news/background-memo-on-the-winners-of-the-european-inventor-of-the-year-2006-awards/203002.article. Accessed 22 Dec 2014
- Shalon D, Smith SJ, Brown PO (1996) A DNA microarray system for analyzing complex DNA samples using two-color fluorescent probe hybridization. Genome Res 6:639–645 ArticleCASPubMedGoogle Scholar
- Lopez M, Mallorquín P, Vega M (2002) Microarrays y biochips de DNA, Informe de vigilancia tecnológica (DNA microarrays and biochips, technological surveillance report).Genoma España/CIBT-FGUAM Google Scholar
- Daudén E (2007) Farmacogenética II. Métodos moleculares de estudio, bioinformática y aspectos éticos (Molecular study methods, bioinformatics and ethical aspects). Actas Dermosifiliogr 98:3–13 ArticlePubMedGoogle Scholar
- Alba R, Fei Z, Payton P et al (2004) ESTs, cDNA microarrays, and gene expression profiling: tools for dissecting plant physiology and development. Plant J 39:697–714 ArticleCASPubMedGoogle Scholar
- Lin SM, Johnson KF (2002) Methods of microarray data analysis II. Springer Science & Business Media, Berlin BookGoogle Scholar
- Wang Z, Gerstein M, Snyder M (2009) RNA-Seq: a revolutionary tool for transcriptomics. Nat Rev Genet 10:57–63 ArticleCASPubMedPubMed CentralGoogle Scholar
- Malone JH, Oliver B (2011) Microarray, deep sequencing and the true measure of the transcriptome. BMC Biol 9:34 ArticleCASPubMedPubMed CentralGoogle Scholar
- Fernández AI, Óvilo C, Fernández A et al (2008) Luces y sombras del análisis de expresión génica utilizando microarrays. Un ejemplo en cerdo ibérico (Lights and shadows of gene expression analysis using microarrays. An example Iberian pig.) ITEA 104:99–105 Google Scholar
- Liu L, Li Y, Li S et al (2012) Comparison of next-generation sequencing systems. J Biomed Biotechnol 2012:251364 PubMedPubMed CentralGoogle Scholar
- Costa V, Angelini C, De Feis I et al (2010) Uncovering the complexity of transcriptomes with RNA-Seq. J Biomed Biotechnol 2010:853916 ArticlePubMedPubMed CentralGoogle Scholar
- Santos CA, Blanck DV, de Freitas PD (2014) RNA-seq as a powerful tool for penaeid shrimp genetic progress. Front Genet 5:298 ArticlePubMedPubMed CentralGoogle Scholar
- Oshlack A, Robinson MD, Young MD (2010) From RNA-seq reads to differential expression results. Genome Biol 11:220 ArticleCASPubMedPubMed CentralGoogle Scholar
Acknowledgments
This work was supported by grants of the Junta de Castilla y León ref. GRS1047/A/14, GRS1189/A/15, and BIO/SA73/15; and by the project “Efecto del Ácido Retinóico en la enfermedad alérgica. Estudio transcripcional y su traslación a la clínica,” PI13/00564, integrated into the “Plan Estatal de I + D + I 2013–2016” and cofunded by the “ISCIII-Subdirección General de Evaluación y Fomento de la investigación” and the European Regional Development Fund (FEDER).
Author information
Authors and Affiliations
- Department of Clinical Biochemistry, University Hospital of Salamanca, Paseo de San Vicente 58, 37007, Salamanca, Spain Ignacio San Segundo-Val
- Salamanca Institute for Biomedical Research (IBSAL), Salamanca, Spain Ignacio San Segundo-Val & Catalina S. Sanz-Lozano
- Department of Microbiology and Genetics, University of Salamanca, Salamanca, Spain Catalina S. Sanz-Lozano
- Ignacio San Segundo-Val